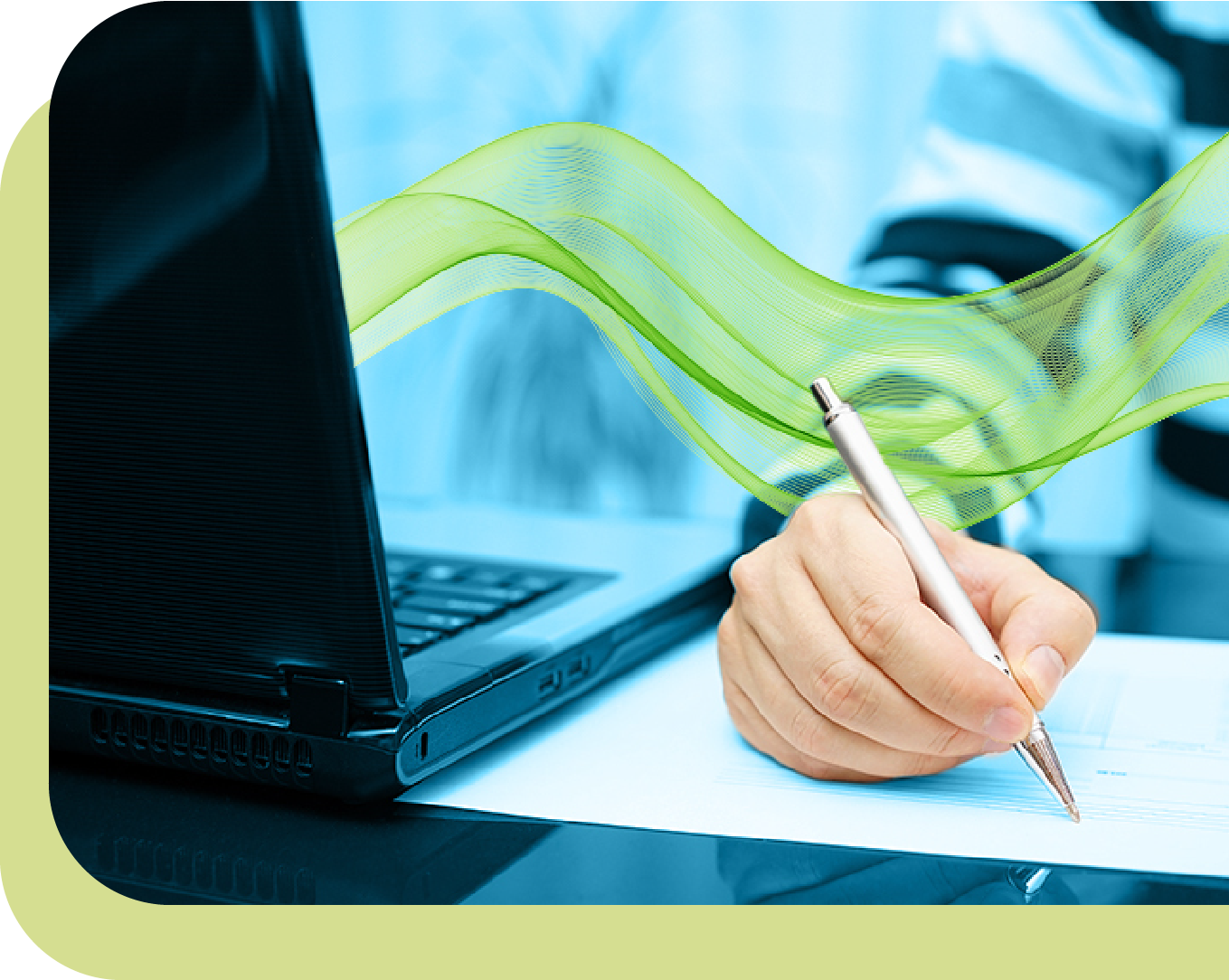
Our Research
The Virtual Learning Lab is using Algebra Nation as the initial test bed for its research. Algebra Nation is part of Math Nation. It was initially developed to help students pass the high-stakes Algebra 1 End-of-course (EOC) Assessment in basic algebra required by the Florida Department of Education for high school graduation. Launched in 2013 by the University of Florida’s Lastinger Center for Learning and Study Edge in response to a mandate that all Florida students must pass algebra, Algebra Nation was quickly adopted by teachers looking for innovative instructional resources. It is now used in every district in Florida, and is expanding into other states.
The research of the VLL encompasses the development of a video recommendation system for Algebra Nation using a combination of unsupervised, supervised and reinforcement learning, causal inference and evaluation of Virtual Learning Environments (VLEs), sensor-free detection of student affect and engagement, methods to estimate student ability, validity and fairness of assessment in VLEs, natural language processing applications to VLEs, and fairness of AI for VLEs . The team of researchers of the VLL include faculty, post-docs and graduate students from University of Florida, University of Colorado – Boulder, and Arizona State University.
Resources related to the Virtual Learning Lab’s research (code and data), can be found on Open Science Framework.
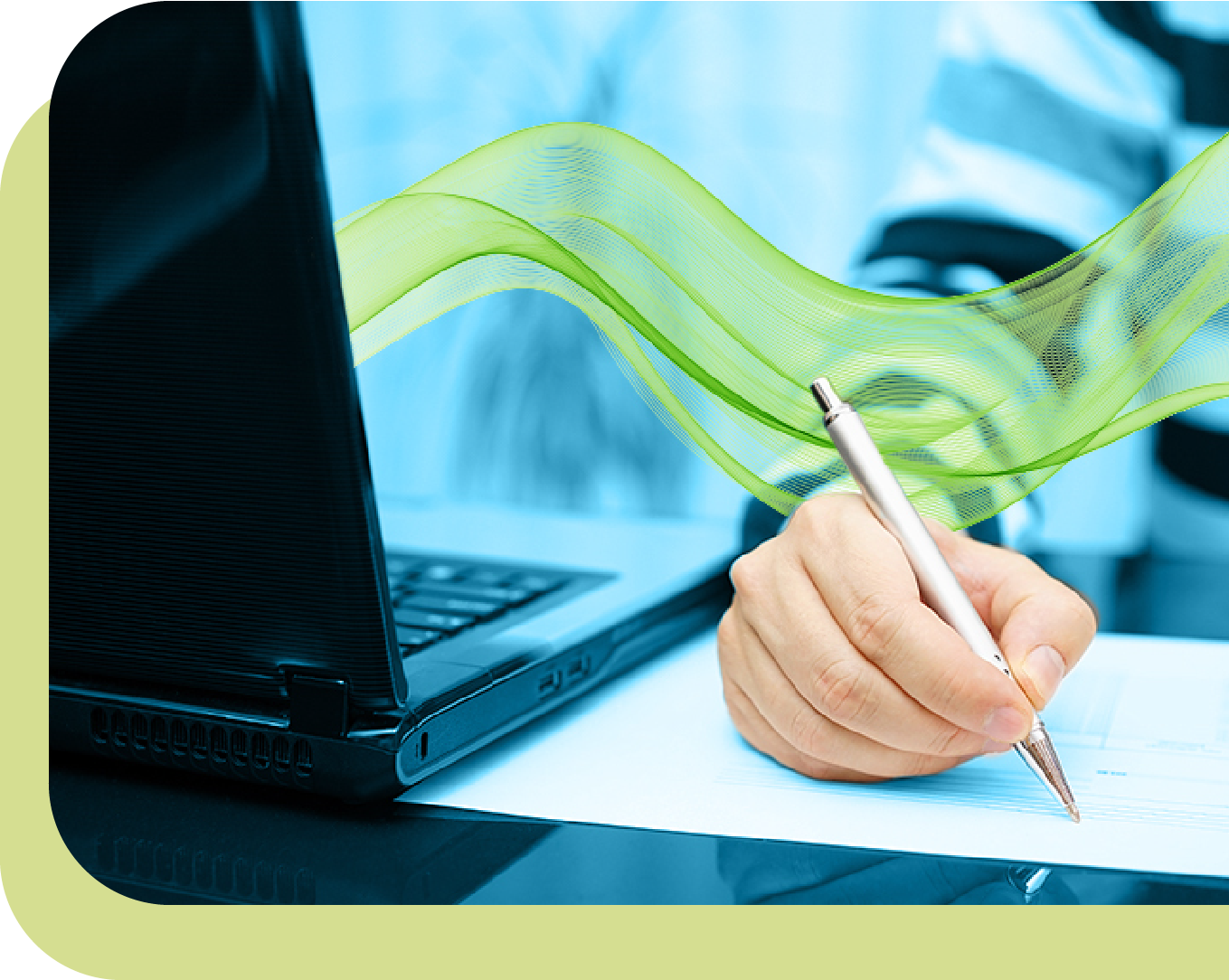
Publications by Topic
Recommendation Systems in Virtual Learning Environments
Leite, W. L. , Kuang, H., Jing,Z., Xing, W., Cavanaugh, C., Huggins-Manley, A. C. (2022).
The relationship between self-regulated student use of a virtual learning environment for algebra and student achievement: An examination of the role of teacher orchestration, Computers & Education, 191.
https://doi.org/10.1016/j.compedu.2022.104615
Leite, W. L., Kuang, H., Shen, Z., Chakraborty, N., Michailidis, G., D’Mello, S., & Xing, W. (2022). Heterogeneity of Treatment Effects of a Video Recommendation System for Algebra. In: Proceedings of the Ninth ACM Conference on Learning @ Scale (L@S’22), p.1-3, New York City, NY, USA: ACM, https://doi.org/10.1145/3491140.3528275
Leite, W. L., Roy, S., Chakraborty, N., Michailidis, G., Huggins-Manley, A. C., D’Mello, S. K., Faradonbeh, M. K. S., Jensen, E., Kuang, H. & Jing, Z. (2022). A novel video recommendation system for algebra: An effectiveness evaluation study. In LAK22: 12th International Learning Analytics and Knowledge Conference (LAK22), March 21–25, 2022, Online, USA. ACM, New York, NY, USA, https://doi.org/10.1145/3506860.3506906
Chakraborty, N., Roy, S., Leite, W. L., Faradonbeh, M. K. S., & Michailidis, G. (2021). The effects of a personalized recommendation system on students’ high-stakes achievement scores: A field experiment. The 14th Conference on Education Data Mining, June 29th – July 2nd Paris, France.
Causal Inference and Evaluation of Virtual Learning Environments
Leite, W. L., Xing, W., Fish, G., & Li, C. (in press). Teacher Strategies to Use Virtual Learning Environments to Facilitate Algebra Learning During School Closures. Journal of Research on Technology in Education. https://doi.org/10.1080/15391523.2022.2110335
Leite, W. L., Jing, X., Kuang, H., Kim, D. & Huggins-Manley, A. C. (2021). Multilevel mixture modeling with propensity score weights for quasi-experimental evaluation of virtual learning environments. Structural Equation Modeling. https://doi.org/10.1080/10705511.2021.1919895
Mitten, C., Collier, Z. K., & Leite, W. L. (2021). Online Resources for Mathematics: Exploring the Relationship between Teacher Use and Student Performance. Investigations in Mathematics Learning, 1-18. https://doi.org/10.1080/19477503.2021.1906041
Kim, D., Lee, Yongseok, Leite, W. L., Huggins-Manley, A. C. (2020). Exploring student and teacher usage patterns associated with student attrition in an open educational resource-supported online learning platform. Computers & Education, 156, https://doi.org/10.1016/j.compedu.2020.103961
Leite, W. L. , Cetin-Berber, D. D., Huggins-Manley, A. C., Collier, Z. K., & Beal, C. R. (2019). The relationship between Algebra Nation usage and high‐stakes test performance for struggling students. Journal of Computer Assisted Learning, 35, 569–581. https://doi.org/10.1111/jcal.12360
Niaki, S. A., George, C. P., Michailidis, G., & Beal, C. R. (2019). Investigating the Usage Patterns of Algebra Nation Tutoring Platform. Proceedings of the 9th International Conference on Learning Analytics & Knowledge – LAK19. https://dl.acm.org/doi/pdf/10.1145/3303772.3303788
Sensor Free Detection of Student Affect and Engagement
Jensen, E., Hutt, S., & D’Mello, S. K. (2019). Generalizability of Sensor-Free Affect Detection Models in a Longitudinal Dataset of Tens of Thousands of Students. In M. Desmarais, C. F. Lynch, A. Merceron, & R. Nkambou (Eds.), The 12th International Conference on Educational Data Mining (pp. 324–329). https://files.eric.ed.gov/fulltext/ED599213.pdf
Hutt, S., Grafsgaard, J., & D’Mello, S. K. (2019). Time to Scale: Generalizable Affect Detection for Tens of Thousands of Students across An Entire School Year. Proceedings of the ACM CHI Conference on Human Factors in Computing Systems (CHI 2019). New York: ACM. https://dl.acm.org/doi/pdf/10.1145/3290605.3300726
Methods to Estimate Student Ability
Jensen, E. (g), Umada, T., Hunkins, N.C., Hutt, S., Huggins-Manley, A. C., & D’Mello, S. K. (2021). What you do predicts how you do: Prospectively modeling student quiz performance using activity features in an online learning environment. Proceedings of the 11th International Learning Analytics and Knowledge Conference (LAK21) in Irvine, CA. https://doi.org/10.1145/3448139.3448151
Xue, K., Huggins-Manley, A. C., & Leite, W. L. (2021). Semi-supervised Learning Method to Adjust Biased Item Difficulty Estimates Caused by Nonignorable Missingness in a Virtual Learning Environment. Educational and Psychological Measurement. https://journals.sagepub.com/doi/full/10.1177/00131644211020494
Xue, K., Huggins-Manley, A. C., & Leite, W. L. (2020). Semi-supervised Learning Method for Adjusting Biased Item Difficulty Estimates Caused by Nonignorable Missingness under 2PL-IRT Model. In: A. N. Rafferty, J. Whitehill, C. Romero, & V. Cavalli-Sforza (eds). Proceedings of The 13th Conference of Educational Data Mining. https://educationaldatamining.org/files/conferences/EDM2020/papers/paper_217.pdf
Natural Language Processing applications to VLEs
Banawan, M., Shin, J., Balyan, R., Leite, W. L., & McNamara, D. S. (2022, June). Math Discourse Linguistic Components (Cohesive Cues within a Math Discussion Board Discourse). In Proceedings of the Ninth ACM Conference on Learning@ Scale (pp. 389-394).
Balyan, R., Arner, T., Taylor, K., Shin, J., Banawan, M., Leite, W. L. & McNamara, D. S., (2022). Modeling one-on-one online tutoring discourse using an accountable talk framework. In A. Mitrovic and N. Bosch, editors, Proceedings of the 15th International Conference on Education Data Mining, pp. 477–483, Durham, United Kingdom, Education Data Mining Society.
Shin, J., Balyan, R., Banawan, M., Leite, W. L., McNamara, D. (upcoming). Combining Discourse Analysis and Theory-driven Natural Language Processing: Revealing Distinct Patterns of Pedagogical Communication in Algebra Tutoring.
Shin, J., Balyan, R., Banawan, M., Leite, W. L., McNamara, D. (2021). Pedagogical Communication Language in Video Lectures: Empirical Findings from Algebra Nation. 2021 Meeting of the International Society of Learning Sciences. https://repository.isls.org/bitstream/1/7484/1/323-329.pdf
Banawan, M., Balyan, R., Shin, J., Leite, W. L., McNamara, D. (2021). Linguistic Features of Discourse within an Algebra Online Discussion Board. The 14th Conference on Education Data Mining, June 29th – July 2nd Paris, France. https://educationaldatamining.org/EDM2021/virtual/static/pdf/EDM21_paper_89.pdf
Validity and Fairness of Assessment in VLEs
Huggins-Manley, A. C., Beal, C. R., D’Mello, S. K., Leite, W. L., Cetin-Berber, D. D., Kim, D., & McNamara, D. S. (2019). A commentary on construct validity when using operational virtual learning environment data in effectiveness studies. Journal of Research on Educational Effectiveness, 12, 750-759. https://doi.org/10.1080/19345747.2019.1639869
Fairness of AI for VLEs
Xing, W., Li, C., & Leite, W. (2022). AlgebraNation Dataset: Demographics-Enriched Data to Support Fair Educational Machine Learning. Proceedings of Fairness, Accountability, and Transparency in Educational Data (FATED 2022) Workshop.
https://fated2022.github.io/assets/pdf/FATED-2022_paper_Xing_AlgebraNation_Dataset.pdf
Li, C., Xing, W., & Leite, W. (2022). Building socially responsible conversational agents using big data to support online learning: A case with Algebra Nation. British Journal of Educational Technology, 53(4), 776-803.
https://doi.org/10.1111/bjet.13227
Li, C., Xing, W., & Leite, W. L. (2022). Toward building a fair peer recommender to support help-seeking in online learning. Distance Education, 43(1), 30-55.
https://doi.org/10.1080/01587919.2021.2020619
Li, C., Xing, W., & Leite, W. L. (2022). Do Gender and Race Matter? Supporting Help-Seeking with Fair Peer Recommenders in an Online Algebra Learning Platform. Proceedings of 12th International Learning Analytics and Knowledge Conference (LAK22).
https://doi.org/10.1145/3506860.3506869
Katz, D., Huggins-Manley, A. C., & Leite, W. L. (in press). Personalized online learning, test fairness, and educational measurement: Considering differential content exposure prior to a high stakes end-of-course exam. Applied Measurement in Education.
Li, C., Xing, W., & Leite, W. L. (2021). Yet Another Predictive Model? Fair Predictions of Students’ Learning Outcomes in an Online Math Learning Platform. Proceedings of the 11th International Learning Analytics and Knowledge Conference (LAK21). https://doi.org/10.1145/3448139.3448200
Li C., Xing W., Leite W. (2021) Using Fair AI with Debiased Network Embeddings to Support Help Seeking in an Online Math Learning Platform. In: Roll I., McNamara D., Sosnovsky S., Luckin R., Dimitrova V. (eds) Artificial Intelligence in Education. AIED 2021. Lecture Notes in Computer Science, vol 12749. Springer, Cham. https://doi.org/10.1007/978-3-030-78270-2_44